"AI in healthcare isn’t about replacing clinicians—it’s about augmenting our expertise. By understanding its capabilities and limitations, we can harness AI to enhance patient care, improve diagnostics, and shape the future of medicine responsibly."
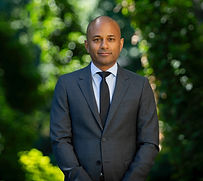
Dr. Andres Jimenez MD
Unit #2
Generative AI For Clinicians: Healthcare Use Cases
Generative AI is revolutionizing healthcare by augmenting clinical decision-making, predictive diagnostics, and patient engagement. This unit explores how deep learning models and neural networks are transforming medical imaging, risk prediction, and clinical workflows, often matching or surpassing human performance. From AI-assisted radiology and retinal scans predicting cardiovascular disease to large language models excelling in differential diagnoses, we examine both the opportunities and risks of AI in modern medicine. Clinicians will gain a foundational understanding of how AI systems function, where they excel, and where they fall short, equipping them to critically engage with these innovations for better patient outcomes.
Lecture (8 min)
Textbook Chapter
We encourage you to watch the lecture above first, then read through the chapter text before attempting the Case Scenario and Quiz below.
Case Scenario #2
Quiz #2
Educational Objectives
-
Evaluate the role of generative AI in medical imaging, predictive diagnostics, and clinical decision support, assessing its impact on diagnostic accuracy and workflow efficiency.
-
Analyze the advantages and limitations of AI-driven diagnostic tools in Radiology, Dermatology, and Gastroenterology, considering real-world case studies and implementation challenges.
-
Compare the predictive capabilities of AI with traditional diagnostic methods, particularly in detecting hidden biomarkers from non-traditional sources such as retinal scans and EKGs.
-
Assess the effectiveness of AI in clinical decision-making by interpreting benchmark comparisons between AI models, medical students, and board-certified physicians.
-
Investigate the ethical and clinical implications of AI integration in electronic health records (EHRs), particularly in decision support systems like the "Green Button" functionality.
-
Critique the impact of AI-driven patient self-diagnosis, identifying both the empowerment potential and risks associated with non-clinician use of AI-generated medical insights.
-
Examine the strategies healthcare institutions use to address AI-related challenges, such as over-sensitivity in imaging, AI confidence scoring, and workflow adaptation.
-
Synthesize best practices for responsible AI adoption in clinical settings, incorporating lessons from real-world hospital implementations and pilot programs.
-
Formulate strategies for integrating AI-driven tools into clinical workflows while mitigating the risks of over-reliance, clinician resistance, and patient misinterpretation.
-
Apply knowledge of AI advancements to propose innovative ways to enhance diagnostic accuracy, patient care, and interdisciplinary collaboration in healthcare settings.