"Just as we rely on rigorous clinical trials to validate medical interventions, we must apply the same disciplined approach to AI in healthcare. Hypothesis testing ensures we don’t just adopt AI—we verify its reliability, mitigate risks, and refine its applications for safer, more effective patient care."
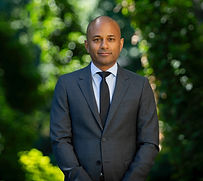
Dr. Andres Jimenez MD
Unit #3
Generative AI For Clinicians: Hypothesis Testing
In this unit, clinicians will explore the essential role of hypothesis testing in the responsible application of Generative AI in healthcare. Just as in clinical research, using AI requires setting clear objectives, defining measurable success criteria, and iterating through controlled trials. This section delves into key concepts such as prompt engineering, structured outputs using XML and JSON, and advanced techniques like few-shot and chain-of-thought prompting to refine AI responses. Clinicians will also learn strategies to mitigate biases, ensure data privacy in compliance with HIPAA, and critically evaluate AI-generated insights against established clinical guidelines to maintain accuracy and reliability in patient care.
Lecture (7 min)
Textbook Chapter
We encourage you to watch the lecture above first, then read through the chapter text before attempting the Case Scenario and Quiz below.
Case Scenario #3
Quiz #3
Educational Objectives
-
Apply hypothesis testing methodologies to evaluate the reliability, accuracy, and compliance of Generative AI applications in clinical workflows.
-
Analyze the risks associated with deploying AI-generated content in healthcare, including potential inaccuracies, biases, and HIPAA compliance concerns.
-
Design and implement structured success criteria for AI-driven clinical solutions, ensuring measurable, specific, and clinically relevant benchmarks.
-
Develop effective prompt engineering techniques, including structured XML tagging and chain-of-thought prompting, to optimize AI-generated responses for clinical use.
-
Critically evaluate AI documentation accuracy through blinded validation studies and iterative hypothesis testing methodologies.
-
Assess the impact of Human-in-the-Loop approaches in AI performance evaluation, refining AI outputs through clinician oversight and feedback.
-
Identify best practices for ensuring patient data privacy and HIPAA compliance when integrating AI into clinical settings.
-
Formulate strategies to mitigate AI hallucinations and improve AI-generated clinical documentation using data-driven refinements.
-
Interpret AI-generated outputs in radiology, diagnostics, and clinical decision-making, balancing AI assistance with human expertise.
-
Synthesize insights from AI hypothesis testing to establish ethical and evidence-based AI deployment strategies in healthcare.