"As clinicians, we rely on accuracy—yet AI can generate false but convincing information. Understanding and mitigating hallucinations isn’t just a technical skill; it’s essential for ensuring AI enhances, rather than compromises, patient care."
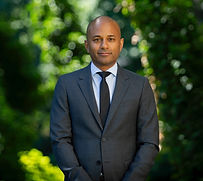
Dr. Andres Jimenez MD
Course Units:
Unit #5
Generative AI For Clinicians: Dealing with Hallucinations & Accuracy
The challenge of hallucinations in Generative AI is one of the most pressing issues clinicians must navigate as they integrate AI into medical practice. This unit explores how Large Language Models generate information, why they sometimes produce false yet convincing responses, and the potential risks in clinical decision-making. It covers essential mitigation strategies, including factual grounding, iterative refinement, retrieval-augmented generation, and parameter tuning, all aimed at improving AI reliability in healthcare. By understanding these methods, clinicians can critically assess AI-generated outputs, minimize misinformation, and leverage AI effectively while maintaining patient safety and ethical responsibility.
Lecture (10 min)
Textbook Chapter
We encourage you to watch the lecture above first, then read through the chapter text before attempting the Case Scenario and Quiz below.
Case Scenario #5
Quiz #5
Educational Objectives
-
Define hallucinations in generative AI and explain their significance in the clinical setting.
-
Analyze the probabilistic nature of large language models (LLMs) and evaluate how it contributes to hallucinations.
-
Differentiate between various causes of hallucinations, including lack of contextual awareness, inadequate training data, and probabilistic output generation.
-
Apply mitigation strategies such as Chain-of-Thought verification, Best-of-N verification, and Iterative Refinement to reduce hallucinations in AI-generated outputs.
-
Assess the role of AI model parameters, including temperature settings and Top-P/Top-K sampling, in controlling the balance between creativity and factual accuracy.
-
Evaluate the effectiveness of Retrieval-Augmented Generation (RAG) in improving AI accuracy, particularly in dynamic clinical environments.
-
Design AI guardrails that enforce compliance with clinical guidelines while preventing speculative or unsupported outputs.
-
Measure AI performance using key evaluation metrics such as sensitivity, specificity, F1 scores, and qualitative assessment techniques.
-
Critique the ethical implications of AI inaccuracies in healthcare, including patient safety, bias, and accountability.
-
Develop a framework for integrating AI-driven decision support tools into clinical workflows while ensuring transparency, reliability, and ethical responsibility.